Research
Professional Goal
Discovering, developing, applying, and advancing the theory of interpretable Bayesian statistical methods to quantify evidence in data relevant to important societal questions, such as infectious disease modeling, efficient utilization of multi-source data in global health, cost-efficient evidence accumulation in clinical trials, understanding bilateral trade flow pattern among countries (in general network time series), drug response prediction in precision medicine, and physical activity analysis (NHANES).
Research Experience So Far
Global Health Research
​We are developing Bayesian methods to analyze disease burden using multi-source data from numerous low and middle-income countries (LMICs). I co-lead a significant global health initiative to improve cause-specific mortality estimates for children under age 5. We have shown that mortality estimates in LMICs, often based on verbal autopsies (VAs), are significantly biased. In fact, the magnitude of bias varies across VA algorithms, countries, and age groups. To address this, we are continuing to develop Bayesian transfer learning methods to correct these biases, enhancing the VA-based cause-specific mortality estimates on a global scale.
Efficient and More Valid Statistical Decision Making
My PhD research on the replication crisis highlighted that Bayesian tests, rather than classical frequentist tests, can effectively quantify evidence to support true null hypotheses. However, their standard implementations often fail to provide strong evidence, limiting their use in clinical trials and related fields. We tackled this issue in a series of works. Initially, I proposed the Modified Sequential Probability Ratio Test to reduce the average sample size required at a specified significance level and power. I then extended this to a class of Bayesian tests with non-local priors, allowing for faster evidence accumulation for both competing hypotheses. In my latest publication, I introduced the Bayes factor function to summarize hypothesis test outcomes comprehensively. This permits the definition of Bayes factors in various important contexts, including linear models and goodness-of-fit tests, and provides explicit evidence in the data for different effect sizes.
Modeling of Structures in Complex Data
-
Penalized linear regression is essential in high-dimensional statistics for managing numerous predictors. To improve its performance with additional external information indicating predictive power and sparsity patterns among predictors, we propose the Structure Adaptive Elastic Net (SA-Enet). This framework incorporates external information by varying penalization strengths for regression coefficients, focusing on group and covariate-dependent structures. We analyze the risk properties of the resulting estimator and extend the state evolution framework, used in the approximate message-passing algorithm, to the SA-Enet for theoretical analysis. Our findings show that the finite sample risk of the SA-Enet estimator matches the theoretical risk predicted by the state evolution equation. The SA-Enet, particularly with informative group or covariate structures, outperforms methods like Lasso, Adaptive Lasso, Sparse Group Lasso, Feature-weighted Elastic Net, and Graper. We demonstrate SA-Enet's effectiveness in analyzing chronic lymphocytic leukemia data from molecular biology and precision medicine.
-
We aimed to assess the significance of modeling network structure in a time series and focused on bilateral trade flows among 29 countries in the apparel industry from 1994 to 2013. In this context, nodes represent countries, and edges represent trade volumes between country pairs. With trades absent in 30% of country pairs, this is an example of a zero-inflated directed network time series. We reformulated it into a paired directed network time series of trade occurrences and volumes, and suggested a joint mechanism for both since they involve the same countries. We introduce the Hurdle Network Model (Hurdle-Net), which employs a latent dynamic shrinkage process for efficiently modeling zero-inflated directed network time series. The model includes several innovative components:
-
I​t handles zero inflation in edge weights through a hurdle model.
-
It uses node-specific latent variables to measure contributions from the latent network dependence.
-
In the presence of an edge, it posits a monotonic increasing relationship between the probability of an edge and its expected weight. Specifically, it employs the generalized logistic function as a general link function to jointly model the two network time series.
-
It applies a dynamic shrinkage process prior on latent positions to capture structures in the latent dynamic evolution of network dependence.
-
-
The National Health and Nutrition Examination Survey (NHANES) conducted by the CDC and the National Center for Health Statistics collects data on the nutrition and health of the US population. Recent samples from 2003 and 2005 include high-frequency physical activity data obtained via hip-worn accelerometers. While this data is often summarized by a single daily activity measure, it is valuable to analyze the functional shape of the activity over time and the distributional aspects of the data.
Motivated by this, we study scalar-on-distribution regression for instances where subject-specific distributions or densities are the covariates related to a scalar outcome via a regression model. In practice, only repeated measures are observed from those covariate distributions. Common approaches first use these to estimate subject-specific density functions, which are subsequently used as covariates in standard scalar-on-function regression. We propose a simple and direct method for linear scalar-on-distribution regression that circumvents the intermediate step of estimating subject-specific covariate densities. We show that one can directly use the observed repeated measures as covariates and endow the regression function with a Gaussian process prior to obtain a closed form or conjugate Bayesian inference. Our method subsumes the standard Bayesian non-parametric regression using Gaussian processes as a special case, corresponding to covariates being Dirac-distributions. The model is also invariant to any transformation or ordering of the repeated measures. To our knowledge, this is the first theoretical study on Bayesian regression using distribution-valued covariates. We propose numerous extensions including a scalable implementation using low-rank Gaussian processes and a generalization to non-linear scalar-on-distribution regression. Through simulation studies, we demonstrate that our method performs substantially better than approaches that require an intermediate density estimation step, especially with a small number of repeated measures per subject.
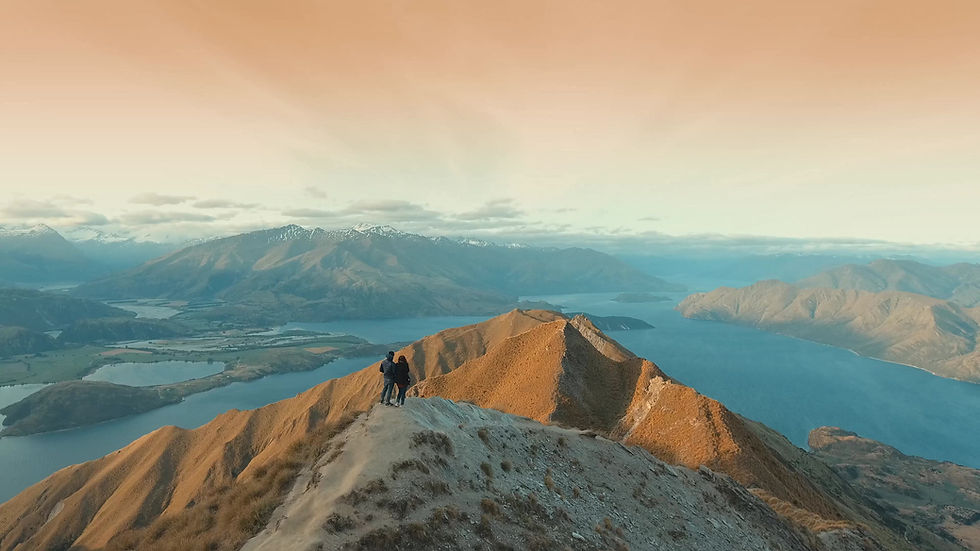